The LLM size Obsession and the future of chatbots building
As the landscape of chatbots and large language models (LLMs) rapidly evolves, there has been a significant focus on increasing model size and data set scale, driven by the belief that bigger models yield better performance. However, size alone does not guarantee effectiveness, prompting the exploration of hybrid architectures and advanced integrations that mark a new era in technology development.
Model Size Race
If you take a closer look, it becomes apparent that this obsession with securing big funding rounds is not always in the best interest of founders. By focusing on raising large amounts of capital, they risk overlooking the fundamental aspect of growing their business and making it profitable. This one-dimensional focus on the next funding round can distract from building a solid foundation and achieving product-market fit.
Historically, the development of LLMs has emphasized scale, with the assumption that bigger models perform better. OpenAI’s GPT-3, featuring 175 billion parameters, set a benchmark for diverse tasks, including text generation and complex translations. GPT-4 expanded this to 1 trillion parameters, pushing performance further. Yet, the sheer size of these models demands significant computational and data resources, making them expensive to operate. Deploying GPT-3 and GPT-4 involves high costs. To address these challenges, OpenAI launched GPT-4o, a streamlined GPT-4 variant, reducing costs while maintaining performance. This marks progress towards more economically viable LLMs without sacrificing output quality. Other models like Google’s PaLM 2 and Anthropic’s Claude 3, though smaller, show that high performance doesn’t require extreme sizes and can avoid issues like output bias. This highlights the necessity for more advanced solutions than just increasing size.
By examining these examples, it becomes clear that the future of LLMs lies in innovative architectures and integrations rather than sheer scale.
Hybrid Architectures: A Path Forward
To address the challenges associated with the sheer size of large language models (LLMs), the future of chatbots and LLMs is veering towards hybrid architectures. These systems combine multiple models and techniques to enhance performance and reliability, ensuring more accurate and contextually relevant outputs. In the following sections, I will highlight some of the more popular hybrid approaches.
Retrieval-Augmented Generation (RAG)
Retrieval-Augmented Generation (RAG) models represent a significant advancement in the field of chatbots and LLMs by integrating retrieval mechanisms that fetch pertinent information, which is then used to generate responses. This approach allows for the provision of detailed, accurate answers, particularly in applications such as customer support. For instance, a customer support chatbot using RAG can access specific product information or past customer interactions to provide precise solutions, thereby improving the quality of support. If a customer inquires about the status of an order, the RAG model can retrieve the exact order history and details, ensuring an accurate and timely response.
RAG models excel in delivering contextually relevant answers by combining the strengths of retrieval-based and generation-based systems. In educational settings, for example, a tutoring chatbot can use RAG to pull up relevant study materials or previous lesson summaries, offering students precise and tailored assistance. Additionally, in technical support scenarios, a RAG-based chatbot can retrieve detailed troubleshooting guides or historical data on similar issues, providing users with step-by-step solutions that address their specific problems.
Leveraging Vector Databases
An emerging trend in enhancing the capabilities of chatbots and LLMs is the use of vector databases. These databases store data in high-dimensional vectors, enabling more efficient and accurate retrieval of information based on semantic similarity. This approach can significantly improve the performance of LLMs by facilitating faster and more relevant data retrieval. For example, in a customer service scenario, a chatbot can use vector databases to quickly find and provide solutions to customer issues based on past interactions and stored knowledge, even if the exact wording of the query differs from previous records. This method ensures that the chatbot can offer precise and contextually relevant responses, enhancing the overall customer experience.
Additionally, leveraging vector databases can drastically improve search capabilities within chatbots. Instead of relying solely on keyword matching, these systems can understand and process queries based on contextual meaning, leading to more accurate and relevant search results. For instance, an e-commerce chatbot can use vector databases to recommend products based on a user’s previous purchase history and browsing patterns, providing a more personalized shopping experience. By analyzing the semantic similarity between current queries and past interactions, the chatbot can offer tailored product suggestions that closely match the user’s interests and needs. This advanced capability not only improves customer satisfaction but also increases the likelihood of repeat purchases, benefiting the business.
Enhancing Autonomy with Integrations and Authentication
The integration of LLMs and chatbots with external systems marks a significant advancement in their autonomy and functionality. These integrations enable chatbots to perform complex tasks beyond generating responses, significantly enhancing their utility. For instance, a chatbot connected to an email system can autonomously manage communications, such as sorting emails, flagging important messages, and even drafting replies based on previous interactions. Additionally, integrating with a contacts database allows the chatbot to update contact information, schedule meetings, and send follow-up reminders. Authentication plays a key role in these integrations, ensuring that only authorized users can access sensitive data and perform specific actions. For example, an office assistant chatbot can manage an executive’s emails and contacts, ensuring that only verified personnel can instruct the chatbot to send emails or update contact information, thus maintaining security and privacy. This blend of integration and authentication makes chatbots powerful tools for enhancing productivity and efficiency in professional settings.
Model Steering, Multi-Modal Approach, and Dynamic Pipelines
An innovative component in the evolution of chatbots and LLMs is the use of model steering mechanisms, which preprocess prompts to determine the best-suited model to handle a query before producing a response. This approach is particularly effective for specialized queries, ensuring that each question is directed to the most appropriate model for accurate and relevant answers. For instance, math-related questions can be routed to computational engines like Wolfram Alpha.
Additionally, a financial chatbot can employ steering mechanisms to route investment-related queries to a financial analysis model, ensuring that users receive precise and informed financial advice. This multi-modal approach enhances the chatbot’s ability to handle a wide range of topics expertly. By integrating different models and databases, chatbots can offer specialized knowledge and insights, vastly improving their utility and effectiveness across various domains.
Dynamic pipelines further enhance this capability by allowing the model to understand a request and create a list of tasks to complete a complex query. For example, if a user asks a travel chatbot to plan a trip, the dynamic pipeline can break down the request into tasks such as booking flights, reserving hotels, and creating an itinerary. This ensures that the chatbot can manage and execute multiple tasks seamlessly, optimizing the performance and accuracy of responses provided by intelligent virtual assistants.
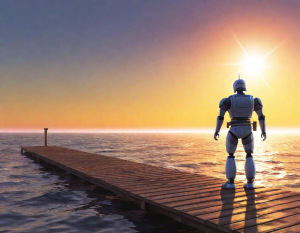
The Future
This multi-faceted approach will lead to smarter, more efficient, and more reliable AI solutions, opening doors to a wide array of applications across different industries. As these technologies continue to evolve, they will empower entrepreneurs to develop cutting-edge solutions that meet the diverse needs of users, heralding a new era of AI-driven innovation and growth. Furthermore, the use and adoption of these advanced LLMs in enterprises and SMBs will revolutionize how businesses operate. By leveraging AI-driven tools, companies can enhance their productivity, streamline processes, and provide better customer experiences. The integration of LLMs into business workflows will enable personalized interactions, improved decision-making, and greater overall efficiency. As a result, both large enterprises and SMBs will benefit from the transformative potential of AI, driving innovation and competitiveness in the marketplace.
Final Thoughts
This is an exciting time for entrepreneurs in the field of chatbots and LLMs. As we move beyond the obsession with model size, there are immense opportunities to innovate with hybrid architectures, vector databases, integrations, and model steering mechanisms. These advancements promise to drive the creation of new startups, both vertically and horizontally, in this rapidly growing category. The future of LLMs lies not in sheer scale but in the sophistication of their design and their ability to integrate seamlessly with various systems.